Insights from J+D Forecasting.
Expert strategies to overcome the inherent inaccuracy of long range pharma forecasting.
Is accuracy ever achievable within long range planning?
When it comes to long-term forecasting, the words of the Greek philosopher Heraclitus, “the only constant in life is change”, have particular significance. We only need to consider recent global events to realize that long-range forecasting is inherently imprecise and unpredictable. Instead, forecasters are better served by identifying opportunities and assessing risks and planning for a broad spectrum of possible future scenarios. In this blog post, we’ll dive deeper into why long-range forecasting is an inaccurate process and look at three strategies forecasters can use to manage these challenges.
Understanding long-range forecasting as inherently inaccurate.
The greatest challenge with long-range forecasting is the interplay of a complex web of variables. It is the forecaster’s responsibility to anticipate uncertainty. While it is fairly straightforward to create a highly accurate short-term forecast, longer-term planning makes it much harder to predict the impact of future changes. Let’s take a quick look at a few critical factors which can significantly impact the accuracy of long-range forecasts.
Discover our Forecasting Solutions.
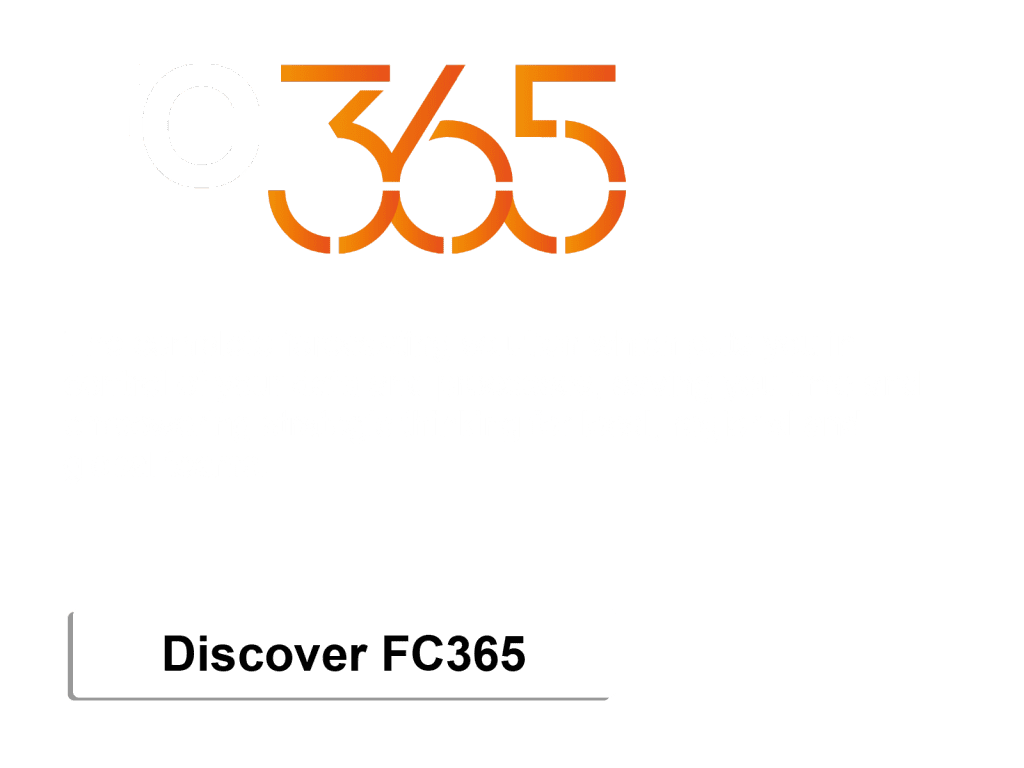
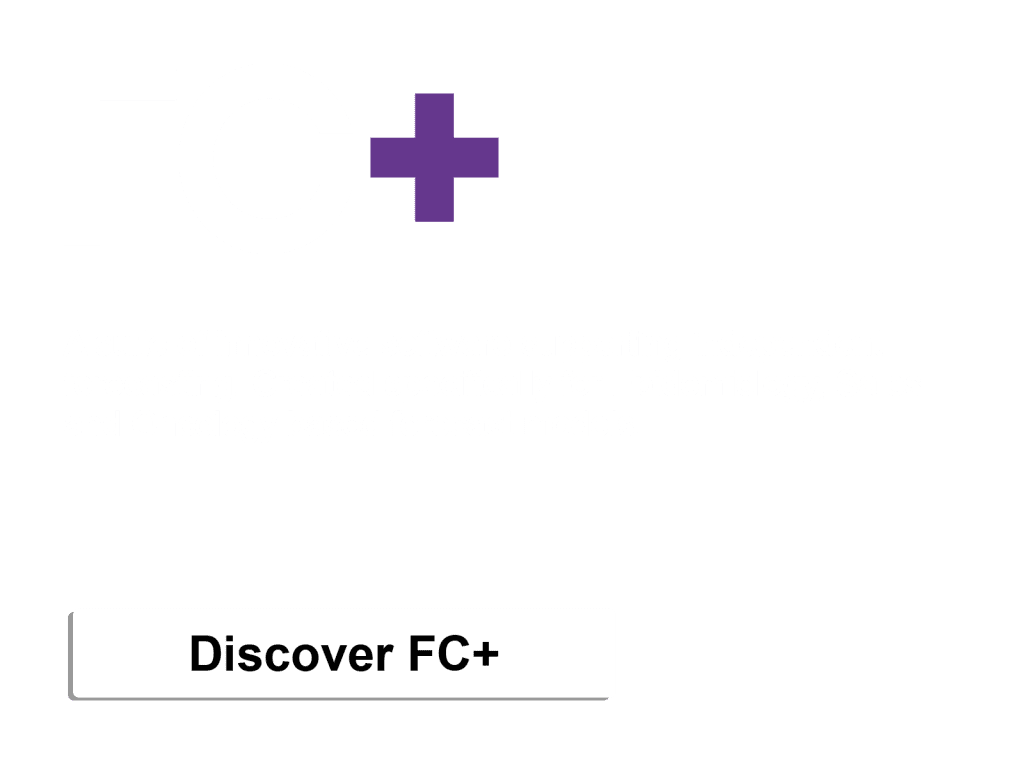
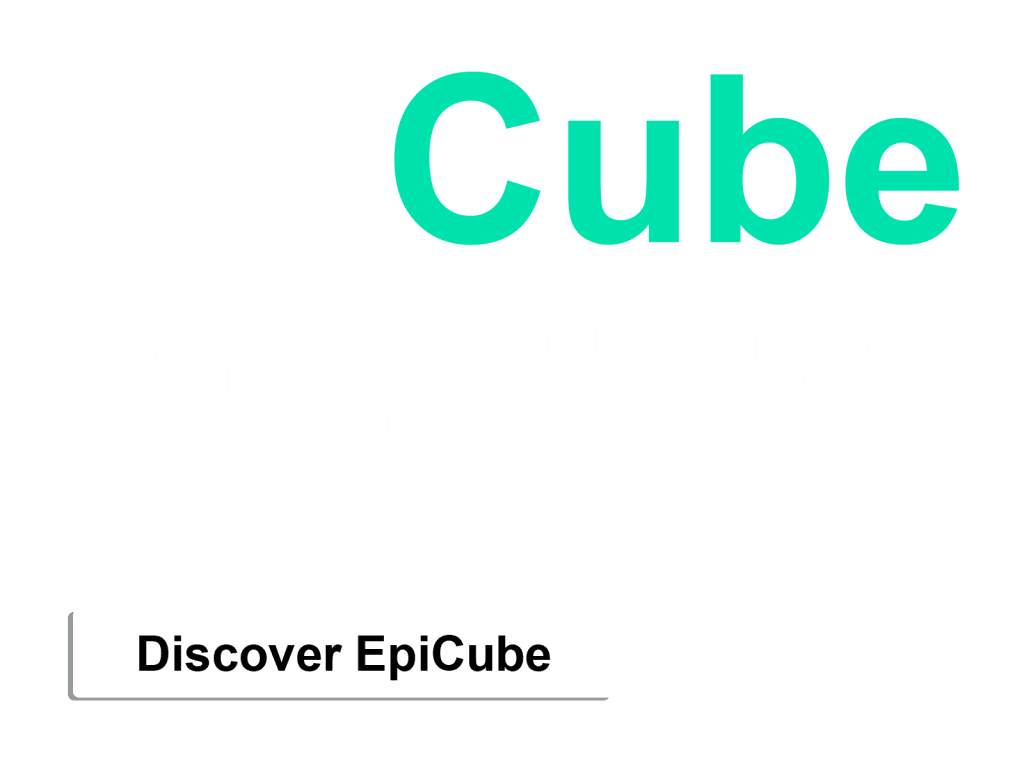
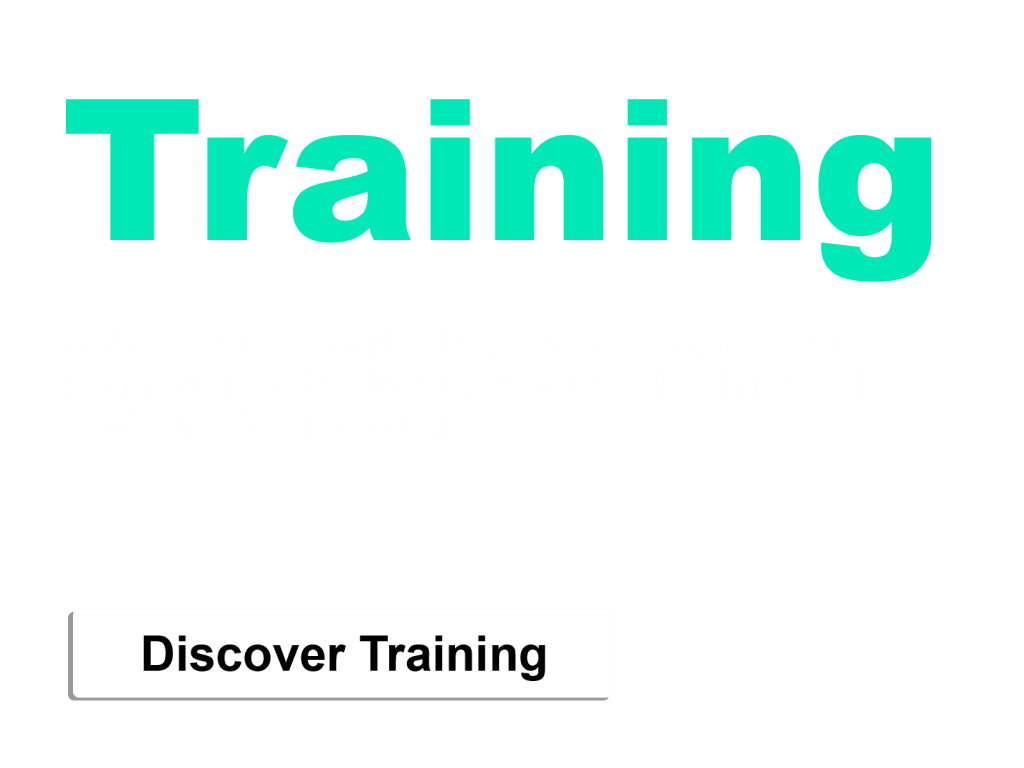
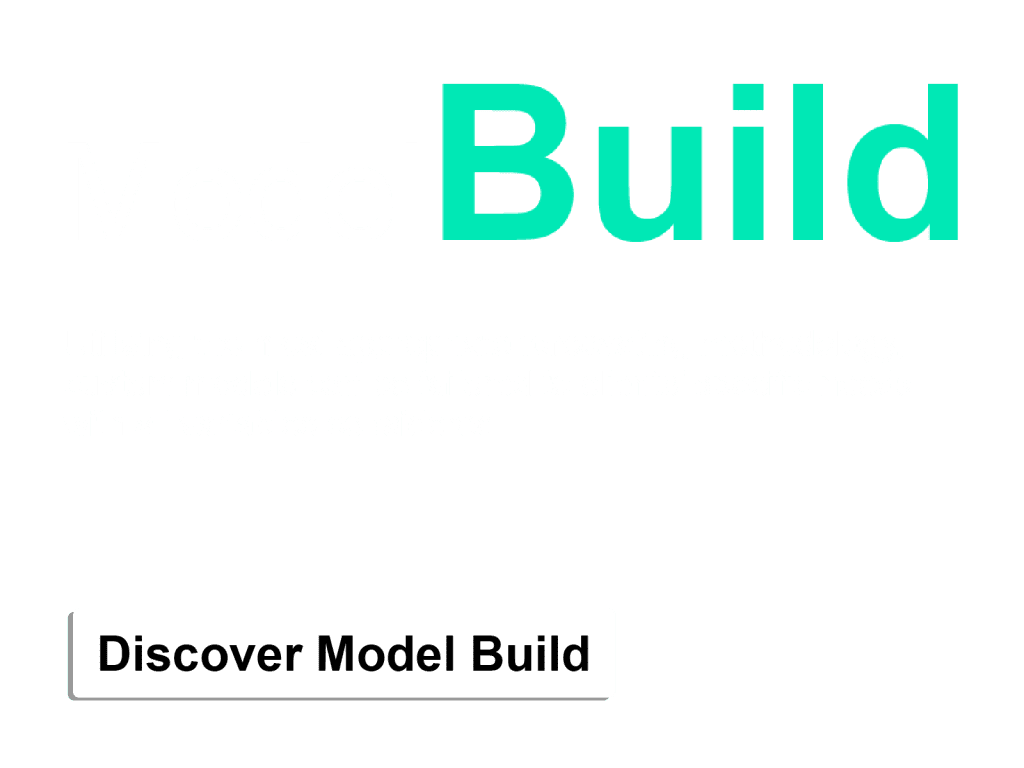
Greater potential for uncertainty
The further out we need to forecast, the greater the potential is for unpredictable events to erode accuracy. In the case of a chronic disease like asthma, there is little variability at the market level since patient numbers remain relatively stable year on year.
The complexity lies in the difficulty of developing and launching new products for these types of diseases. According to this
report by the Congressional Budget Office, just 12 percent of drugs entering clinical trials are approved by the FDA.
With studies showing estimated average R&D costs per new drug ranging from less than $1 billion to more than $2 billion, forecasters have the challenging responsibility of trying to predict which competitive products might enter the market and how successful they might be, in addition to a web of other variables.
Political and internal motivations.
One more controversial factor that forecasters should be aware of is any potential internal or political motivations that may impact the accuracy of the forecast. The internal stakeholders of a certain drug may push for an overly optimistic forecast in order to secure ongoing funding for their research. Alternatively, companies may be driven by a desire to convince shareholders that they have a strong pipeline for new products.
For example, one company we worked with pushed internally to develop a female sexual dysfunction drug with very strong forecasts, positioning it as the next Viagra. The product was sold on to another company who later discontinued development when they discovered it was not a viable option to bring to market.
Opinion-based data.
A final variable to consider is the type of data being used for long-range forecasting and how this information is captured. Reliance on market research can be a limiting factor, especially since physicians tend to overestimate their likelihood to prescribe particular products.
Considering how to obtain evidence-based rather than opinion-based data is one way to increase the accuracy of forecasting.
Three Strategies for Better Long-Range Forecasting.
1. Methodology.
Choosing the right modeling methodology is the first key to better long-range planning. At J+D Forecasting we advocate event-based forecasting. This enables you to model the impact of factors that fall outside of the scope of historical data. For example, this might be the impact of a competitor’s product launch at an unknown point in the future. With this approach, you can easily modify key data points to understand the impact of a variety of possible future scenarios.
Rather than focusing on accuracy above anything else, forecasters should aim to present a range of possible future scenarios. A solid model which has the sophistication to capture the full range of factors impacting the forecast enables forecasters to adapt their output response to stakeholder requests. For example, this type of model might be used to demonstrate the steps a company would need to take to reach a particular financial goal with a new drug launch.
2. Identify variability.
Focusing on variability can help forecasters better identify risks and opportunities. To enhance long-term forecasting, the key is to begin with robust data and identify the main sources of variability. Investing in accurate data-gathering for these areas of variability helps to drive better assumptions and understand the risks and opportunities of a particular scenario.
The next step is to run multiple scenarios which capture the inherent uncertainties of the future competitive landscape, defining the most likely, worst and best-case outcomes.
3. Clear communication.
Last but not least, forecasters should also consider how they communicate long-range forecasts to stakeholders and decision-makers. Forecasts should empower an organization to execute strategic long-range business plans with confidence and clarity. Long-range forecasts should be designed to be as user-friendly as possible. This can be achieved by filtering and presenting only the key information that stakeholders need and quoting ranges rather than precise numbers for greater accuracy.
This type of presentation requires a mindset shift for forecasters away from the laser focus on accuracy toward a broader top-level approach. Stakeholders should be able to come away with a clear understanding of a particular project's upside and downside potential, which can directly enhance their executive decision-making processes.
Final Thoughts.
01
The foundation of long-range forecasting is a solid and transparent model which can be easily adapted and improved as new data becomes available.
02
Rather than focusing on accuracy, long-range forecasters should strive to understand where risks and opportunities lie.
03
Focusing on variability helps to identify risks and opportunities efficiently and effectively.
04
Communicating these risks and rewards clearly to decision-makers empowers them to act strategically and decisively in accordance with the data available.
1. Methodology.
Choosing the right modeling methodology is the first key to better long-range planning. At J+D Forecasting we advocate event-based forecasting. This enables you to model the impact of factors that fall outside of the scope of historical data. For example, this might be the impact of a competitor’s product launch at an unknown point in the future. With this approach, you can easily modify key data points to understand the impact of a variety of possible future scenarios.
Rather than focusing on accuracy above anything else, forecasters should aim to present a range of possible future scenarios. A solid model which has the sophistication to capture the full range of factors impacting the forecast enables forecasters to adapt their output response to stakeholder requests. For example, this type of model might be used to demonstrate the steps a company would need to take to reach a particular financial goal with a new drug launch.
2. Identify variability.
Focusing on variability can help forecasters better identify risks and opportunities. To enhance long-term forecasting, the key is to begin with robust data and identify the main sources of variability. Investing in accurate data-gathering for these areas of variability helps to drive better assumptions and understand the risks and opportunities of a particular scenario.
The next step is to run multiple scenarios which capture the inherent uncertainties of the future competitive landscape, defining the most likely, worst and best-case outcomes.
3. Clear communication.
Last but not least, forecasters should also consider how they communicate long-range forecasts to stakeholders and decision-makers. Forecasts should empower an organization to execute strategic long-range business plans with confidence and clarity. Long-range forecasts should be designed to be as user-friendly as possible. This can be achieved by filtering and presenting only the key information that stakeholders need and quoting ranges rather than precise numbers for greater accuracy.
This type of presentation requires a mindset shift for forecasters away from the laser focus on accuracy toward a broader top-level approach. Stakeholders should be able to come away with a clear understanding of a particular project's upside and downside potential, which can directly enhance their executive decision-making processes.
Final Thoughts.
01
The foundation of long-range forecasting is a solid and transparent model which can be easily adapted and improved as new data becomes available.
02
Rather than focusing on accuracy, long-range forecasters should strive to understand where risks and opportunities lie.
03
Focusing on variability helps to identify risks and opportunities efficiently and effectively.
04
Communicating these risks and rewards clearly to decision-makers empowers them to act strategically and decisively in accordance with the data available.
our team is ready
To Help
If you have a forecasting challenge to discuss, contact us and one of our experienced consultants will be in-touch to arrange a free consultation.
Your information will be used in accordance with our privacy notice.
More Insights from J+D.

Forecasting Considerations throughout the Pharmaceutical Product Lifecycle
Forecasting plays a vital role throughout the product lifecycle. We explore the considerations forecasters must

Tailoring Forecasting Approaches to Each Stage of the Pharmaceutical Lifecycle
From market assessment in the early stages to adapting forecasts based on specific product and

Mastering Stakeholder Alignment for Effective Pharmaceutical Product Launch
Launching a pharmaceutical product demands collaboration across global, regional, and country teams. Balancing stakeholder input
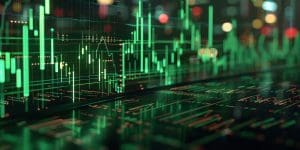
Client-Focused Pharma Forecasting: Building Models that Shape Strategy
In the pharmaceutical world, forecasting plays a crucial role in shaping strategy and ensuring market