At the start of 2023, we reported on the increasing need for pharma to digitalize their processes for more efficient forecasting.
Throughout the year, we have seen an increasing number of large and small pharma companies finally jump on board the technology train as increasing demands and diminishing workforces pushed the need for connected solutions to the fore.
The rapid acceptance and progression of AI during the second half of the year has been a major factor in this, as its potential within drug development was realised.
Let’s explore our pharma forecasting predictions for 2024:
1. Artificial intelligence redefining forecasting
AI and machine learning (ML) are already playing a critical role within the pharmaceutical industry. The best use cases for these technologies are drug discovery, drug manufacturing, diagnostic assistance, and optimizing medical treatment processes.
Following a number of early announcements from big pharma who had already collaborated with or acquired AI technologies (AstraZeneca partnered with Nvidia, Sanofi implemented Plai), news slowed as many big pharma battened down the financial hatches during the uncertain mid-year period. We are now seeing renewed partnership news, with two deals in two days by Phenomic AI announcing a $500million deal with Boehringer Ingelheim and a collaboration with Astellas-owned cell therapy biotech Xyphos Biosciences, showing that the appetite for adoption of this technology is still strong.
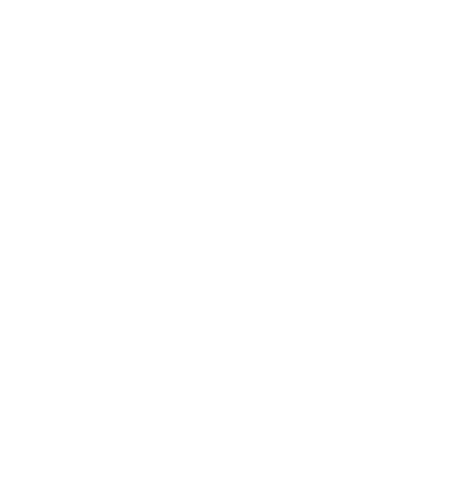
The implications of these technological advances need to be considered by the forecaster to help guide strategy. Hypothetically, early diagnosis and treatment will have a fundamental impact on most progressive diseases and given that the current R&D strategy is to develop drugs targeted at late stage, hard to treat patient cohorts, the consequences are potentially significant.
Earlier diagnosis of diseases has implications on patient outcomes, therefore change patient distribution across lines of treatment or severity of disease. The forecaster will need to reflect this in their forecast models. The most significant impact is likely to be within oncology with improved 1st line treatment resulting in less focus on next line drug development.

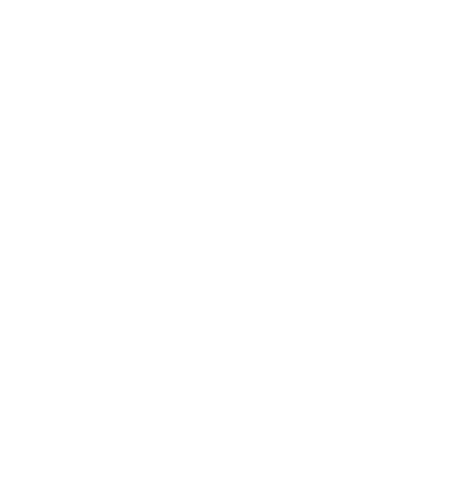
2. Forecasting application outcomes
AI is already being used to predict epidemics, but if we apply the same approach to other disease areas, we can more accurately predict how some diseases will evolve, taking into account demographic, causal, environmental, and socioeconomic factors. We could also, for example, model the impact that AI driven diagnosis has on the progression of diseases!
There are several other potential applications for the use of AI in the development of forecasts, from the use of ML to model the evolution of diseases to create more accurate market sizing, the estimation of peak share of drugs coming to market, as well as the use of NLP (Natural Language Processing) to automatically generate insights.
Improved decision-making: AI-based tools can provide insights and recommendations based on data analysis, enabling pharmaceutical companies to make more informed decisions about product development, market entry and pricing strategies.
Faster and more efficient forecasting: AI-based tools can automate the forecasting process, reducing the time and resources required to generate forecasts.
With enormous potential to revolutionize the way clinical trials are conducted, improving patient selection and speeding up recruitment, AI could lead to significant reduction in time to market and associated R&D costs. This decrease in drug development time and cost will require adjustments to forecast assumptions, namely around probability of success, time to launch and key NPV assumptions.
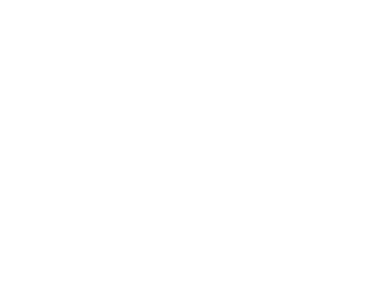
50% reduction in time to market
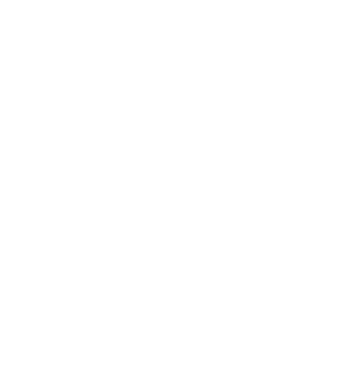
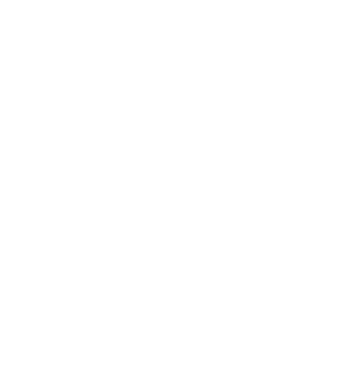
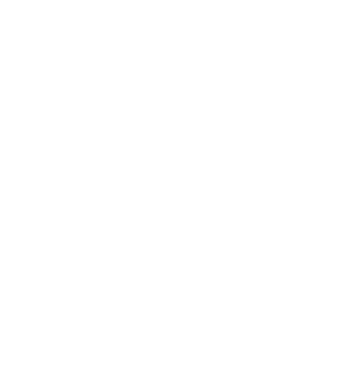
50% reduction in associated costs.
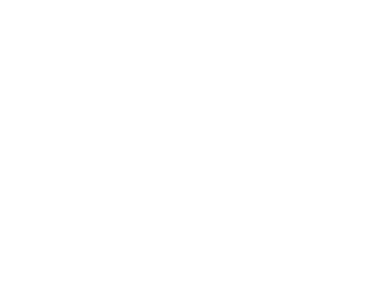
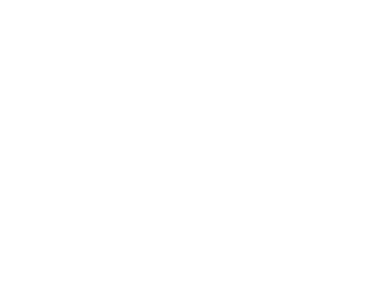
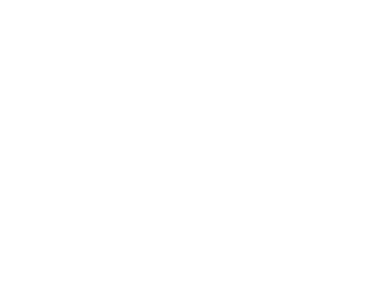
50% reduction in time to market
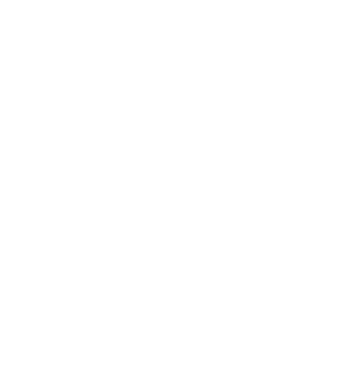
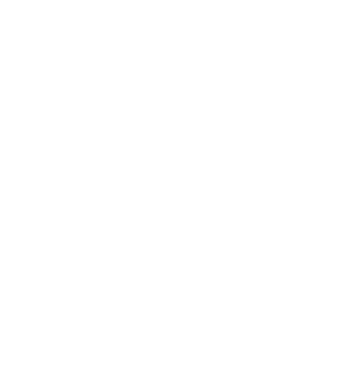
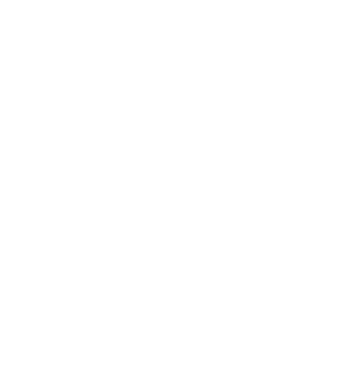
50% reduction in associated costs.
3. Business streamlining across key functions
The challenges that pharmaceutical companies face are ever-changing and not necessarily universal, varying widely by therapy area, region, or type of business. For big pharma, the pressure on pricing continues through regulation and generic substitution, as well as the need to fill the pipeline with innovative compounds. For evolving small Biotech, the challenging economic environment is increasing scrutiny from investors to deliver value.
During 2023, many big pharma announced senior leadership changes leading to a shake up across divisions. Others, focussed on the race to market for particular drug lines, or facing loss of exclusivity for leading therapies, saw them streamline divisions resulting in redundancies and office closures. Takeda announced layoffs across the US in a pipeline shake-up to prepare for generic competition to its diabetes blockbuster. Novartis cut 103 positions in clinical operations as part of CEO, Vas Narasimhan’s approach to “enable faster trial recruitment and enhanced trial delivery.”
With sales dropping by nearly two thirds, demand for the Covid vaccine is another factor impacting future decisions for the major players. Pfizer are already planning another $500million in cost cuts in response to the expected decline, with 70% of this cost-realignment impacting R&D investment.
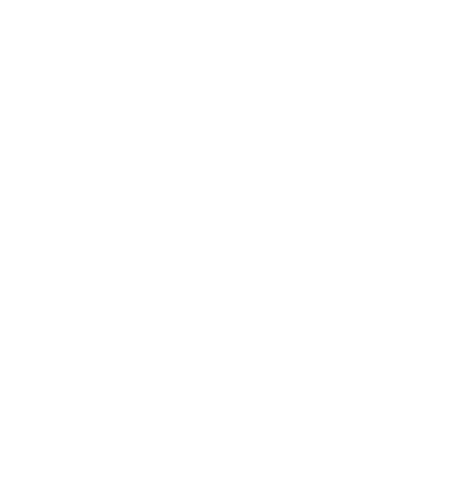
So what is the impact of this streamlining on pharma forecasting teams?
Increased demand for modern solutions.
Continued business streamlining expected throughout 2024 will put pressure on forecasting teams to stay ahead of changes. Driving the need for flexible forecasting software which can easily adapt to changes in business direction and commercial needs. There is no place for disjointed, time-consuming and error-prone forecasting processes that drain expensive business resources. Having access to modern, high-performing tools will become crucial to underpin good strategic and operational decision-making.
Increased demand on NPP teams, model building timeframe reduced.
As pharmaceutical companies start using technology across the broader business functions, the development of drugs will become quicker. Drug discovery – identifying the right compounds with the right profiles for diseases will improve as companies adopt innovative AI technologies. Further efficiencies within clinical trials and data analysis will follow. With AI reducing years of manual work to mere days, lab resources required for drug discovery lessen, inevitably leading to R&D streamlining. The expediting of drug development will demand more from new product planning (NPP) and launch teams, necessitating the build of complex forecast models in weeks rather than months.
Global integration becomes essential.
These digital efficiencies are also removing need for large teams/divisions, resulting in a reduced number of experienced pharmaceutical forecasters within regional or global teams. Less staff will increase reliance on industry-specific forecasting solutions. Advanced capabilities will be demanded of specialist software and connected platforms, and easy global integration and usability will be a must.
Cloud-based technology is gaining popularity as an efficient option for these forecasting teams. It simplifies process management, automates tasks, integrates systems, and enhances collaboration within an organization. The main challenge in adopting a new forecasting system is getting stakeholders to learn a different approach. However, by choosing platforms using familiar Microsoft tools like Excel and Power BI, team members can focus on forecasting instead of spending extensive time training stakeholders on unfamiliar external software.
More importance placed on forecasting tools.
Responsibility for sourcing suitable forecasting solutions is another shift. Procurement or Finance functions are frequently leading RFPs (Request for Pricing) for forecasting solutions and technologies as it moves into focus as an essential function supporting key business decisions across pharma organizations.


4. Advanced visualization capabilities
The utility of data visualization by pharma forecasting teams falls into three main goals: to explore, to monitor and to explain. The ability for forecasting teams to quickly recognize and easily understand patterns can lead to faster and more accurate decision-making for senior stakeholders.
We expect the demand for enhanced visualization to accelerate in 2024 as streamlined teams focus on more complex forecast modelling to include additional countries/therapy lines. Commercial teams under increasing pressure will need flexible analytics tools with advanced visualization capabilities that respond quickly to model changes. Most important will be access to real-time updates and synchronization between forecast models and analytics.
Adoption of connected forecasting platforms can address this demand, where teams can gain valuable insights easily and make data-driven decisions. From standard PowerPoint presentations to detailed reports for day-to-day forecasters, and clear summary dashboards showing KPIs to satisfy senior management needs. All dynamically connected to the underlying models. These solutions enable faster, better, and more strategic decisions to be made by pinpointing key drivers for individual brands/opportunities and across countries/portfolio.
Use of familiar Microsoft based analytical tools such as Power BI facilitates connectivity to an ever-growing list of data sources and objects. Standard or customised forecast reports and dashboards built from this data can then be embedded into the platform solutions, to fully meet clients’ needs. Scenario planning is made easy with the ability to run ‘what if’ virtual scenario analysis. Stakeholders can then explore variables within the forecast and make informed decisions in the room.
Loss of exclusivity (LoE) , an inevitable part of the pharmaceutical industry, requires complex modelling to incorporate biosimilars or generics entering the market and challenging competitive positioning. With a number of big pharma facing LoE in 2024, it will be crucial for commercial teams to have premium analytics tools to visualize the changing market dynamics and inform vital strategic decisions.
5. From In-House to On-Demand Expertise
Over the last few years, we have seen more and more pharma bring their forecasting processes in-house as the need for upskilled teams was realised. Budget previously allocated for outsourced forecasting functions was reassigned to provide specialist forecasting training and software. Equipped with the right models and skills, forecasting teams have become more self-sufficient, bringing efficiencies across a number of planning and commercial functions.
Now, with the reduction in workforces and cost-conscious procurement teams, demand is now increasing for vendors to provide not only specialist software and training, but also support services. On-demand access to professionals, whether for peak periods or to address specific forecasting needs, will increase to expand teams’ capacity and capabilities. Ranging from business analytics to complex model population and development, access to specialized experts as an extension of their team will ensure they have the right resources at the right time.
This is not a simple case of returning to previous forecasting service vendors. The fast, pressured environment of pharma forecasting, with its differing needs along each product lifecycle, requires timely, accurate and expert support. Therefore, vendor teams positioned within local time zones are becoming the preference of big pharma, avoiding the challenges associated with off shoring projects such as lack of relevant industry experience, availability, response times, and frequent changes in personnel.
With this new approach to forecasting support, expect to see less reliance on global data organisations as forecasting teams look for a comprehensive range of support from experts in pharmaceutical forecasting methodologies, advanced experience in process and change management, plus technical expertise. Vendors who will be as much a part of the team as in-house colleagues.
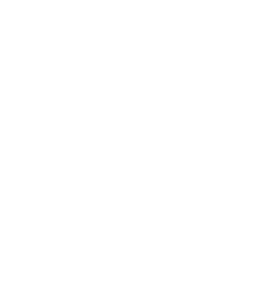
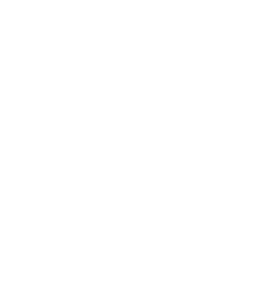
Speak with an expert.